Data Engineer’s Role in the Future of Groceries
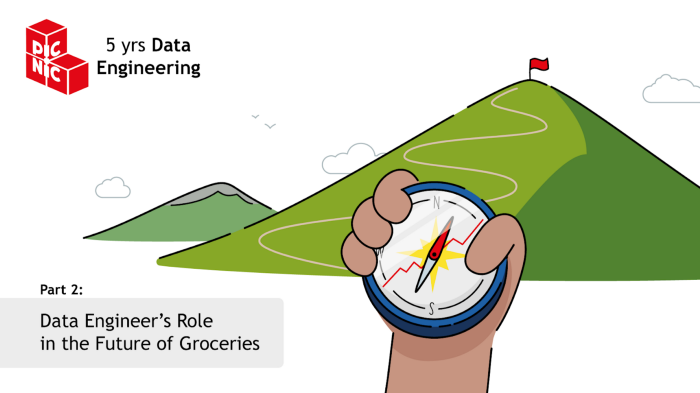
How Data Engineers collaborate with other roles at Picnic? What are some real business challenges solved by using the Data Warehouse? Where next?
This is the second article from five, in a series where we take a deep dive into Data Engineering here at Picnic. This post is dedicated to the business challenges that Data Engineers solve together with other teams, and lays out how we see the road ahead of us.
In years gone by, the milkman did home delivery, evolving from horse-drawn carriages to petrol or diesel vans. Some believe this belongs entirely in the past, but at Picnic we’ve created “Milkman 2.0” — delivering groceries with electric vehicles, reducing food waste, and minimising food miles. And we use data as the compass for navigation while reinventing the concept.
A brief intro to Picnic’s Data Warehouse
Before we dive into what it means to be a Data Engineer at Milkman 2.0, and how we help the business, a quick intro of what Picnic’s Data Warehouse (DWH) is all about:
In my first blog post, Picnic’s Lakeless Data Warehouse, we explore the technologies and architecture of our Data Engineering ecosystem. We talk about why we have a strong preference for structured DWH and how much we value data quality. Also, briefly scratch the surface of Data Vault and Kimball data modeling and the best use cases for each.
The bottom line is that our DWH setup allows us to solve issues in the supply chain, make customers happy, and be environmentally-friendly. The DWH is a source for analysis, KPI reports, Slack updates, visualisations, calibration of operational systems, and machine learning algorithms.

As owners of the DWH, Picnic’s Data Engineers learn about every business area domain, and they help solve the most pressing challenges for the company.
Just like the friendly librarian advising about where to find the latest novel, we support colleagues in getting the data they need quickly and easily.
Data-driven solutions to business challenges using the Data Warehouse
The purpose of our DWH is to allow Business Analysts and Data Scientists to focus on what matters: creating insights and making smart decisions to improve our business.
Below is a shortlist of 10 projects that the Data Engineering team helps to solve with well-structured and high-quality data.
1. Tracing products throughout the supply chain
As a supermarket, we strive to deliver the best and freshest products. Our vegetables, fruits, meats, and fresh bread need to be top-quality.
To make sure this happens, we collect data every step of the way, for each product, from the moment we receive it to the moment we deliver it.
We know where it was stored and for how long, and we know the temperature of the crate during delivery, the speed and bumps it experiences in the electric van, and whether the customer made any complaints (and if so, about what). This is powerful for making sure customers receive their avocados and ice cream in the perfect condition.

2. Eliminating food waste
We place the purchase order to our suppliers after the customer creates their order. Just in time, whenever possible. And when we have to order ahead, our machine learning models rely on the historical data from the DWH to predict the exact amount to order from the supplier.
If the stock is much higher than the demand, there are damaged products, or products aren’t fresh enough, this will potentially generate waste. By being able to track this, Picnic can tackle the root causes of waste generation and continuously improve our stock management practices.
3. Maintaining an efficient supply chain
We carry the optimal assortment of products that customers need in any season, but take great care not to overstock with too many similar products.
Within the app, we collect feedback about which products people want. If enough people request them, we add them to the assortment. And if a product isn’t popular, we remove it quickly to make space in our fulfilment centres.
Whenever we have issues in the supply chain and a product isn’t orderable, we suggest the best alternative. Just to be sure, we carry out extensive A/B tests, all based on data from the DWH.
4. Providing a superb delivery window promise to the customer
We provide a delivery window of just 20 minutes. Compared to the few hours usually given by delivery services, this is very convenient. To make this efficient and scalable, we rely on high-quality data from the DWH to calculate optimal drop times using machine learning.
For example, there’s a big difference in the time it takes for a new driver to deliver to a customer on the third floor of a building without an elevator (carrying three heavy crates) versus an experienced driver delivering to a ground floor property, carrying three light crates.
5. Being sustainable
Picnic is environment-friendly. We operate more than 1,000 electric vehicles. And to go even further, we extend the battery life of our vehicles by using data on all the trips they make, the outside temperature, and the driving conditions at any moment. We analyse how these conditions impact battery range, and use it to plan charges accordingly.
6. Committing to safe driving
A massive amount of data is collected on every trip about acceleration, as well as other driving parameters. This makes it possible to “steer” on the key performance indicators for safe driving. The information raises awareness and transparency, helping build a culture of mindful driving.

7. Responding quickly to customer issues
We support our superstar Customer Success team to respond to customer feedback in minutes. While the agent focuses on connecting with the customer, a machine learning model classifies the customer message in the background.
The algorithm uses historical data to speed up the resolution, and we use Natural Language Processing (NLP) algorithms to make it faster to process the delivery feedback.
8. Building a best-in-class online store
With our app, we’re striving for customers to have an easy and enjoyable three-minute grocery shopping trip. If a feature hinders the user experience, we redesign it — for iOS and Android.
For example, we made a major redesign of the app to create a thumb-friendly app tab bar, after suspecting the new generation of larger phone screens to be changing how our app is used.
To truly understand this, we looked into the data on existing usage, and ran A/B tests. Here, we noticed patterns of “compartmentalised” usage around different kinds of activities. As a result, we split the whole experience into tabs — navigate, search, basket, overview, and profile.
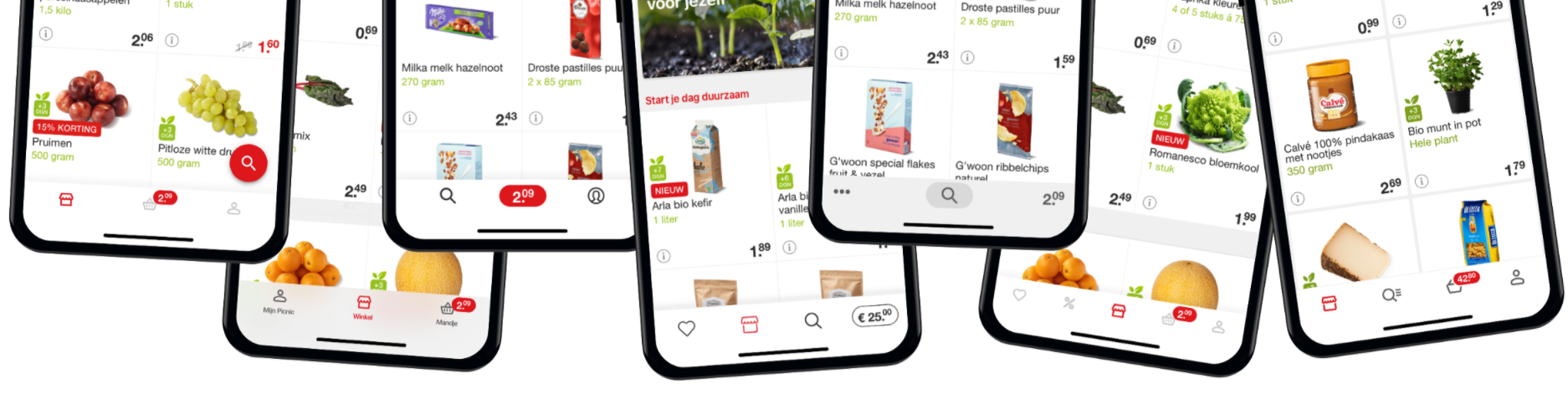
Another example: in 2018, we introduced a seemingly simple change. We moved from a conventional order rating system using five stars, to one using three emojis. For this change, we carefully analysed how the customer will use the new feature.
The emoji system has prompted customers to give 20% more ratings, and 125% more qualitative feedback. At the same time, it helped our Customer Service team to work more efficiently, spending 18% less time dealing with order feedback — and reducing overall workload by 3% per day.
9. Improving operational systems
In addition to improving our business, the DWH helps our Product Owners decide on which features to build. For example, as our homemade Warehouse Management System expands with features for guided flows, the fulfilment team’s Business Analysts can quickly measure improvements and make the business case for certain new features.
10. Coping with unusual times during COVID-19
During Coronavirus times, we had to adapt to daily changes in the supply chain, and we had to scale our systems to handle the increased demand for grocery deliveries.
Picnic opened a new fulfilment centre in Germany within a few weeks. And data helped us quickly ramp up the operation at this new site.
At the same time, we dedicated capacity purely for essential workers to be able to get their groceries at home. This needed a rapid response from our software teams to build a “priority” list feature. Also, required us to use data to manage capacities and get accurate forecasts on orders from this important group — and to fill the rest with regular orders.
Another example is an unusual use case for Picnic’s Data Vault implementation. The government in The Netherlands introduced a regulation overnight which prohibited the sale of alcohol from 20:00 the next day. This included Picnic orders, of course.
Since we always plan our deliveries for the next day, we had to take immediate action, by removing beer, wine, and other alcohol from future orders — as well as the existing paid ones. Naturally, this wasn’t a feature we’d already developed, so we had to think on our feet. We ran some Data Vault processes to capture the latest state across many systems and made an initial assessment of the impact. This example shows the usefulness of the Data Vault in a microservices ecosystem.
Data Engineering at Picnic: What does the role involve?
The most exciting part of my job is that I can quickly see the impact of my work in the physical world. Every project we work on is tangible, and we often know within weeks whether it has been successful or not. This constant feedback loop is a renewable source of motivation for me, as there’s always something to learn that can be done better next time.
Almost every analytics project at Picnic uses data from the Data Warehouse that our team has carefully architected and built. Over the course of a month, a Data Engineer works on at least three projects that will introduce them to different areas of the business.
Working closely with Data Scientists and Business Analysts
We share a lot of skills with Data Scientists and Business Analysts. Our common language is Structured Query Language (SQL), which is the primary language for building data transformations here at Picnic. More than 80% of the business analytics logic is in SQL. We’re also using Python widely — both in production and prototyping.
And besides the hard technical skills, we share good business sense and an ability to communicate. These soft skills are fundamental, as this creates an enjoyable and intellectually-stimulating environment. It also makes sure we’re critical of the challenges we solve, and we constantly raise questions about whether our understanding of the goals is clear.

Our Data Scientists focus on predicting the future with machine learning, while our Business Analysts put their minds to creating insights and making decisions in the present. And our Data Engineers focus on having high-quality data on the past, which the other two roles depend on.
For this, we follow mature Software Engineering principles to build the DWH and all the Extract Load Transform (ELT) processes. Data Engineer’s superpower is Data Modeling. We use frameworks such as Data Vault and Kimball Dimensional Modeling to create order from the chaos.
Working closely with back-end teams
The story wouldn’t be complete without mentioning the awesome work that our back-end teams are doing to maintain data quality. Examples of these systems include Master Data Management, Purchase Order Management, Warehouse Management System (WMS), Master Planning Process, Distribution Runner App, Store, and Accounting.
These systems are the source for all the data in the DWH, and I must say that the overall emphasis on data quality throughout all the services is quite something to behold. In my previous experience, Data Engineers were often left to fix bad data without much help from upstream systems. This couldn’t be more different here at Picnic!

Consumer-Driven Contracts with in-house Picnic systems
The development teams built REST end-points, and generate events according to a schema contract — which minimises the chance of something going wrong. This is also known as Consumer-Driven Contracts.
With REST end-points data, for instance, we use Data Transfer Objects (DTOs). These aggregate and encapsulate data for transfer. DTOs don’t usually contain business logic, but serialisation and deserialisation mechanisms. The fields and their types are defined in the DTO directly in the source system, which is a safeguard against unintentional changes to the response.
Those kinds of issues are caught during development and testing, and rarely reach production or cause issues in the data pipelines. Most of the in-house REST end-points used for the DWH are specifically built for that purpose. They allow for ‘from_timestamp’ and ‘to_timestamp’ parameters, allowing us to incrementally pull data without impacting the performance of an operational system. And the response is streaming — thereby reducing the risk of overloads and out of memory errors.
We heavily use events for analytics, which is complementary to REST end-points. The contract with the producer is expressed in a self-descriptive JSON meta-schema. There, we define the names of the fields, express complex object nesting and constraints. One of the most important constraints is the list of required fields. The schemas are owned by the source system, and the event payload is validated in unit tests if it complies with the schema.
Going the extra mile to achieve high data quality
The measures we have in place sustain high data quality and stable ingestion pipelines to the DWH. But things can still go wrong. And when they do, it’s all hands on deck to find a solution.
Here’s one example, which comes to mind from a few weeks ago:
As a result of a network issue, all events emitted in an hour-long window from our WMS intended for the DWH ended up in a “bad” event stream. We could have quickly recovered the events and loaded them in the DWH, but there was one crucial piece of information missing — the origin warehouse site!
With multiple warehouses and hundreds of events per minute, we could see that a product was picked but didn’t know where. This rendered the data for that window useless. The back-end WMS team came up with a plan for recovering this data from internal logs. After a lot of effort by many people, we managed to restore 100% of the data.
It was inspiring to see everyone going the extra mile. In another environment, people might say “Leave it, it’s not worth recovering one hour of warehouse data. We have big data consisting of billions of events. Why bother for a few thousand?” — and to that, we’d say it’ll impact the reporting for weeks (or months) to come, and it’ll break machine learning models for years. It’s worth the effort to have complete trust in our data.
Continuous growth and improvement: meeting the challenges ahead
We’ve achieved so much in the past five years. But it feels like it’s just the start. The challenges ahead are as hard as ever, and the only way ahead is to level-up. For that, we need more brilliant and motivated people to join us!
Any of the below initiatives could fill another five years by themselves. The most exciting part is that we need to tackle them all — and in fact, many more! It is a nice mix of business, technical and organisational topics.
- ????️ Sustaining a monolith DWH in a microservice environment, and focusing on event processing. One example of a successful implementation of a microservice is our RunnerApp used by drivers for navigation, providing ETA to customers, and registering recyclable returns. With the rise of microservices, the data complexity also increases.
- ???? Scaling up the team and the processes in line with the rapid growth of the company. This includes expanding collaboration on GitHub pull requests with the Tech and Business teams in Picnic.
- ???????? Scaling up advanced training of Business Analysts on SQL and data visualisation skills, to extract even greater value from the Data Warehouse.
- ???? Open-sourcing some of the automation tools that we believe will help the whole Data Engineering community. We aspire to move the Data Engineering discipline closer to the more mature DevOps landscape, as well as delivering value to our business.
- ???? Continuing international expansion, potentially across time zones. I visited Australia and loved it, and it would be awesome if Picnic decides to expand there. To get ready for this, we need to step up our game to report dynamically in the correct timezone. Any Data Engineer who has worked in a global organisation knows what I’m saying here!
- ???? Building a highly-automated fulfilment centre that will serve 150,000 orders per week. It will feature shuttle systems in three temperature zones, and the goal is to deliver the best service at the lowest cost. Such a site will generate a massive amount of data 24/7. It’s estimated that the daily volume from an automated fulfilment centre is equivalent to the data generated for a whole year in a manual fulfilment centre. Long before operations actually start, we’re already generating and analyzing simulation data which we store in the DWH. To learn more about the Picnic & TGW innovation in automation, listen to this podcast (in Dutch) with Frank Gorte and Jan Willem Klinkenberg.
- ???? Improving distribution performance with our data will remain a hot topic for the Data Engineering team. We’ll work hard on improving our battery management, and building advanced predictive maintenance models. At the same time, for international expansion into new markets, having demographics data is key for defining our distribution areas. And for new areas where we don’t have sufficient data on historic drop times, using data on building structure will be very interesting. Solving these challenges requires a DWH which can extract insights from geographic and voxel data.
- ???? Evaluating new innovative value propositions using data. For instance, recently, we started a partnership with DHL to provide a service for parcel returns. We collect return packages from a customer, bring them to our hubs, and from there DHL picks it up. This adds more convenience for our customers, and is a great extension to our services.
- ???? Making online grocery shopping even easier. There are many features in the area of e-commerce that we are exploring. One of which is to make online payments in our app faster, safer and seamless. For this reason, we are developing our own Picnic pay method in partnership with Rabobank, Mastercard and Adyen.
Key takeaways: climbing the highest peaks with our data compass
Data Engineers at Picnic play an essential role in bringing the data produced by Tech teams to Business Analysts and Data Scientists, who make data-driven decisions. We are a multiplier in the company, enabling everyone to find the right analytical data, trust it, and use it responsibly.
Besides the technical challenges, we also focus on working with other teams to promote data governance best practices and improve SQL skills throughout the company. Our Data Warehouse powers a range of decisions that span the whole supply chain. From providing a service that customers love and offering the optimal range of products, to reducing food waste, operating a large fleet of electric vehicles, running efficient warehouses, and maintaining a high-tech eCommerce platform.
At Picnic, we’re climbing a very steep mountain. One step at a time. Each step needs to be taken carefully, to sustain our energy for the long-term. Data Engineers help provide reliable data compass, so the leaders can make solid decisions about which direction each step will take.
As we climb, we also build a road for the rest of the expedition to follow. This structure is key to scaling, and vital for expanding into new markets and different value propositions.
“The summit is what drives us, but the climb itself is what matters.” These words, uttered by Conrad Anker, one of the world’s most accomplished alpinists, perfectly sum up our journey.
This post explored some of our exciting climbing challenges. In the next article, the third in our series, I’ll share how we solved the challenges at the beginning of our climb. It will be full of war stories about starting a Data Team, delivering analytical value to a rapidly growing startup, making impossible choices between urgent and ultra-urgent projects, and learning the hard way. ⛰️
Recent blog posts
We're strong believers in learning from each other, so
our employees write about what interests them.