Picnic 10 years: 2016 — The Milkman Routes
Picnic turns 10 this year! To celebrate such an achievement we will highlight each year in a series of blog posts where you can learn about our successes and our challenges. Last month, our CTO Daniel Gebler reflected on the 10 most important lessons learned throughout our 10 years. This month, we will focus on the second year. 2016 not only was the year of Pokemon Go and Stranger Things, but also the year in which we wanted to prove that we can crack the last mile challenge. To take you through this journey, we will highlight the three key pillars of delivery: the model, the execution and the tech powering it all.
The challenge
Ordering online is rapidly becoming the norm across an increasing number of retail verticals. However, grocery delivery proves to be a different game. Even though the market is growing steadily, there are many reasons for it to be lagging behind: orders are larger (50+ items compared to 2–3 items in non-food categories), margins are lower, delivering perishable and cooled items is challenging. At Picnic, we are building sustainable operations with efficiency at the core of everything we do. This allows us to overcome these challenges and deliver high quality products without a delivery fee. During our second year we realized that we can achieve said goals, only if we approach the last mile in a radically different way.
The model
We can only run efficient grocery operations if we have efficient delivery, and you can only deliver efficiently if you have high customer density. Customer density will grow organically: the more customers we serve, the lower the average shortest distance from one customer to another will be. But, we can also control this by getting customers that are close to one another to place an order that will be delivered at the same time. If two neighbours both place an order, we can still not deliver efficiently if one of them is delivered on Wednesday and the other on Thursday, or one in the morning and the other in the evening.
In 2016, we worked out a model where the number of delivery slots we allow a customer to choose from changes depending on their neighborhoods density. The idea behind this is very similar to that of a bus rather than a taxi: a customer does not get full freedom when the delivery is, but we offer you a selection of slots from which at least one will suit your needs. The trade off is that we can deliver more efficiently and eliminate the requirement of a delivery fee.
Originally, we only offered three one-hour delivery slots per week: depending on where you lived, you and your neighbours would be able to choose for example Monday 16:00–17:00, Wednesday 21:00–22:00, and Friday 18:00–19:00. Very quickly we realised that this was too constrained and we exposed 6 delivery slots per week already in late 2016. Now, with significantly higher density, we can efficiently run our operations in most delivery areas with 7-day a week service while also offering delivery slots from morning to evening, making customers even more satisfied with our product.
The execution
To deliver efficiently, we don’t only need efficient trips, but also efficient drops. We needed to design a vehicle and delivery operations that minimised the time spent parking, unloading large deliveries and getting them to our customers while also allowing our runner to provide high quality service. Moreover, we really wanted this vehicle to be fully electric, even though it has to carry a heavy payload.
As a result, the vehicle we designed not only powers the most efficient distribution system on the planet, but is also the cutest car you will see driving around.
This Electric Picnic Vehicle, or EPV for short, has a couple of very handy features. First of all, it is relatively small, meaning that it is easy to find a parking spot close to where you need to be, and quick to park without blocking other drivers, a commonly heard complaint in the logistics industry. Secondly, it opens from the side, which means that we very efficiently use the space in the vehicle, and it is easy for the runner to find the exact articles to be delivered. Furthermore, the vehicle is accompanied by a nifty piece of software called the Runner App — developed in-house since 2018, this was already identified as a key component to allow efficient execution. The Runner App guides the runner throughout the delivery flow: it seamlessly transitions from a trip overview to navigation, to highlighting which crates need to be delivered, to handling the flow after the delivery has been completed by taking in empty bottles or return packages and trip aftercare. This help not only caused significant reduction of the delivery times, it also allowed us to improve driver safety.
The tech
Now that we have established that in order to achieve success we require short deliveries and short driving times, we must now focus on reaping the benefits by creating efficient planning. Based on a full day plan with many deliveries, we need to divide these deliveries into efficient trips without exceeding vehicle capacity and weight constraints, while also arriving during the delivery window that the customer selected. With this aim, we plan our trips in four steps: we plan the delivery crates we call totes, we estimate how long the delivery will take, we create the trips, and then we determine the vehicle layout.
Planning the delivery totes is closely related to the bin packing problem, a well-studied problem in optimisation literature. However, for us there are a couple of additional objectives that are unique to groceries: we are dealing with multiple temperature zones, a multitude of items, quality sensitive products, contaminating items, among others. We have designed a bag planning algorithm that not only meets the minimum totes required to fulfill the delivery, but also takes into account the fragility of the articles. Therefore, the algorithm won’t plan a bottle of bleach in the same tote as the apples you’ve bought, and given the allocation options left, assigns the item in a manner that is quickest to pick our warehouses.
Once we know how many totes a delivery requires, we can estimate how long the delivery will take. The number of totes of course is a large influence here, but the time of day, forecasted weather conditions, on which floor a customer lives and whether there is an elevator present also plays a deciding factor in the delivery. This is done using machine learning, and you can find the full process described on this blog: Optimal drop times using machine learning.
Next up in the process is trip planning: once we’ve calculated all totes and estimated all delivery duration, we have everything we need to actually create the routes. By modifying a problem known as the Vehicle Routing Problem with Time Windows in optimisation literature to our use case, we were able to build an algorithm that is fully optimised for our urban delivery model. We could not simply use an existing algorithm, but had to adapt this to our unique constraints, including multiple temperature zones, and weights. However, this also comes with opportunities to optimise the approach in ways that generic algorithms are unable to do so. For example, we can subtract scheduled drop time when successive deliveries are close to one another. This is something we face very often, whereas an algorithm designed to plan semitrucks will not benefit from this feature at all. Moreover, we use (meta)heuristics that align with our model since we have significantly shorter routes than traditionally faced in literature, and can use the nature of our time windows to efficiently prune many solutions we know to be infeasible. The algorithm designed in 2016 even achieved multiple world records on benchmark instances that closely resembled the Picnic use case — while performing terribly on longer trips, a trade off we happily made.
The final step in the planning process is a lot simpler — or at least we thought so in 2016. Given a delivery trip, we need to figure out which tote goes where in the EPV. We initially implemented a greedy heuristic which prefers to plan a delivery on a single side of the EPV so the runner does not have to fetch totes from multiple sides during a delivery. Furthermore, it also takes into account that heavy totes balance the EPV if we place them on the bottom creating a safer trip.
Throughout the trip the tote distribution should be similar: it’s not safe driving around when the left side of your EPV is stocked with heavy groceries, and the right side is completely empty. However, as deliveries increased, we realised that there are many more objectives for us to optimise on, but that’s a topic deserving of its own blog post.
Lessons learned
Throughout the years, we have made many iterative improvements to the model, the EPV, and planning algorithms including its framework. We added features to our drop time prediction, additional constraints to our tote planning and business objectives to trip plannings. As these problems became more complex to optimise, we learned two valuable lessons. Firstly, we learned that although direct heuristics allow for quick operational impact, investing the time in generalizing your optimisation problem and setting up a solution using metaheuristics yields a more extendable and maintainable implementation. This allows faster feature delivery as the complexity increases with your operational understanding. Secondly, we took the time to build a simulation framework for all these problems and their interactions. This allows operators and analysts to independently evaluate their new ideas or optimize configurations, unlocking key insights like optimal delivery schemas or vehicle designs. Now, as battery and vehicle technologies improve and our service area grows and customers are located in more rural areas, we also use this simulation framework to plan out the next iteration of our delivery model. When we are no longer limited by vehicle range and delivery times decrease, how should we design our ideal routes, delivery windows and algorithms to serve the customers of the future? We are as eager to find out as you are!
Picnic 10 years: 2016 — The Milkman Routes was originally published in Picnic Engineering on Medium, where people are continuing the conversation by highlighting and responding to this story.
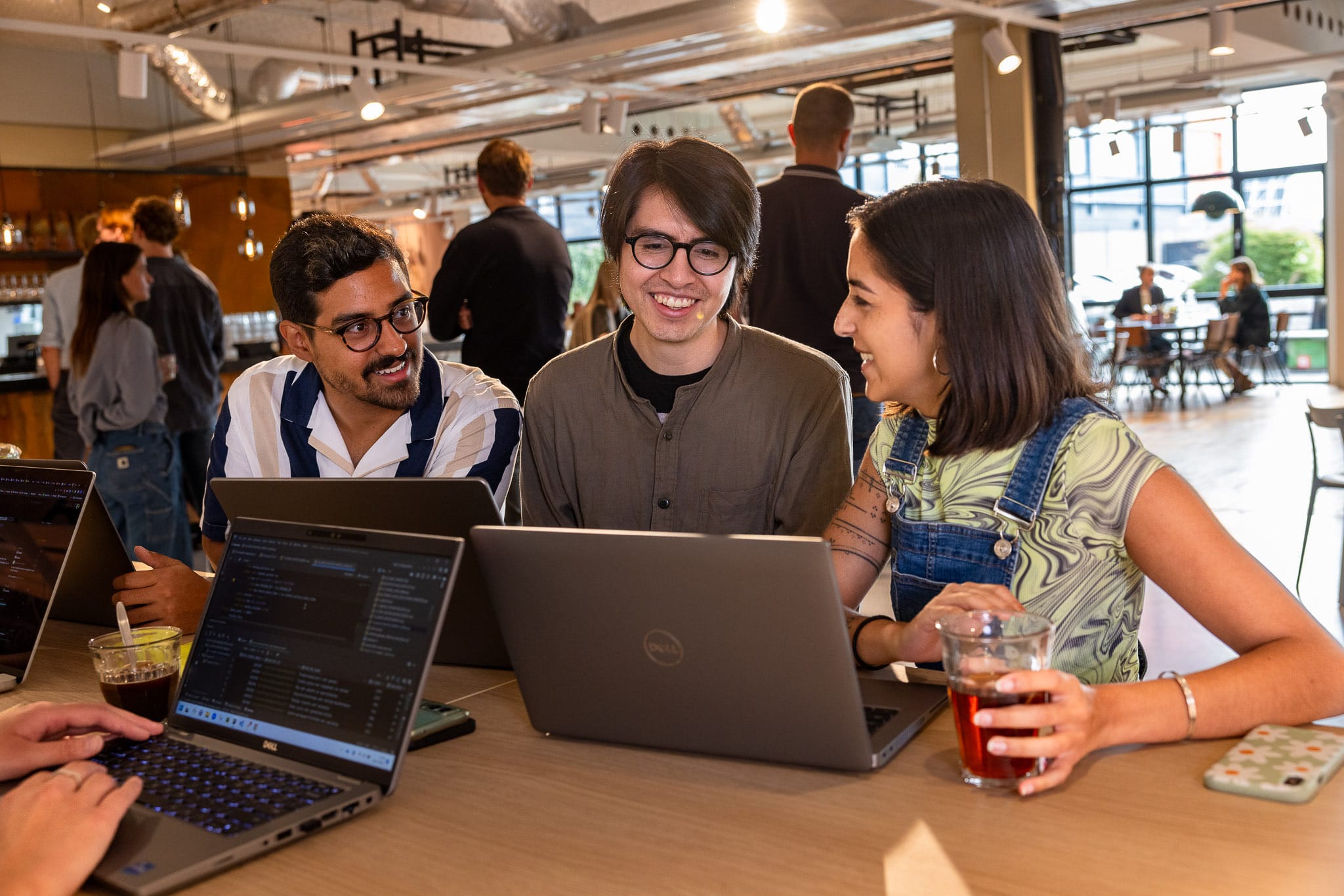